Journal of Political Risk, Vol. 1, No. 5, September 2013.
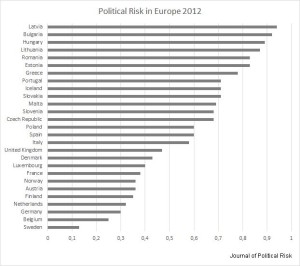
Figure 1: Political Risk in Europe 2012. The index arranges the countries relative to one another. The separate values would change if more cases are added to the sample.
By Stoycho P. Stoychev, Ph.D.
This paper proposes a quantitative index of political risk in Europe, based for the first time on corruption, market distortion, and the shadow economy. It is constructed upon the idea that within a continuum between rule of law and corruption, the levels of political risk vary greatly. Institutional statistical data are used to allow for reliability in time and cross-country comparison. As a proof of reliability, the resulting scores are highly correlated with other applied indices of political risk. A major advantage of the proposed index, however, is that it differentiates between developed countries, which is not possible with existing risk indices.
Introduction
The post-revolution expropriation of foreign companies in Cuba introduced social science to the concept of political risk (Bouchet et al. 2003). Growing interest in the subject from the 1960s to the 1980s was followed by a decline in the 1990s. Political risk seemed redundant to political scientists, because it appeared to be a catchall concept incorporating various aspects of institutional stability, performance, and policy in a single, still superficial term. The qualitative nature of the processes embedded in that broad category hindered quantitative approaches. This caused the abandonment of the concept of political risk and replacement with country risk in the 1980s, and sovereign risk in the decades onwards. Both concepts were much more tangible and empirically measurable. Growing numbers of fiscal and monetary metrics replaced pure political ones in operationalizing political risk, thus placing the analytical tradition in the domain of economics.
The financial crisis and contagion effects between countries in recent years, however, disclosed the relevance of the broader concept of political risk not only in the commercial sector, but in the academic discipline of political science. Corrupt and inefficient governments worldwide proved incapable of providing the desired levels of development to their societies, and the crisis now looks much more political than financial or economic. It is a crisis of biased regulations and biased institutions, both resulting from political ineffectiveness and inadequate policies.
Left nearly at the margin of scientific interest, the notion of political risk remains poorly conceptualized. What is now considered mainstream literature on political risk defines this phenomenon as the probability of financial losses inflicted against foreign investors by active or passive behavior of stakeholders within a political system (Root 1972; Simon 1982; Howell and Chaddick 1994; Roy and Roy 1994; Meldrum 2000; Howell 2001; Brink 2004; McKellar 2010). Thus political risks sometimes appear limited to war, revolution, expropriation, nationalization, confiscation, taxation or contract repudiation. The victims of political risk are then foreign companies or foreign investors in general, which have invested in the respective country and are consequently subject to the sovereign policy of the host government.
These conceptions of political risk could be appropriate for very specific contexts, but in general are limited and biased. First, they are focused on the output of the political system without providing explanation of the mechanisms that have led to specific undesirable outcomes. Understanding the black box of decision-making is crucial for any decent forecast of the likely actions of political stakeholders. Second, events like wars, revolutions and expropriations could cause heavy losses to foreign investors, but they are specific to very unstable regions. Thus, risk-assessing methodologies are insufficiently sensitive to grasp differences among the so-called developed countries, where other problems like corruption and organized crime could cause commensurable financial losses to investors. Third, these investors are merely one among several entities subject to political risk. Local businesses, banks and foremost the local population are also vulnerable.
Good estimation of political risk, along with associated probabilities, causes, and effects, is a major contribution of political science, yet insufficiently developed. These estimations should be based on a more rigorous approach than that currently applied by the risk-rating agencies. The first step is an improved conceptualization of quantified political risk. The purpose of this paper is to provoke a discussion on the topic that leads to a better quantitative understanding of the definition and metrics of political risk.
Conceptualization
The classic Oxford English Dictionary definition of risk is a situation involving exposure to danger. Therefore, political risk follows from a political condition or event exposing the interested party to danger. Within a country, the factors that could produce such conditions or events are twofold. On one hand, the institutional system acts as an environment for political interaction. On the other hand, diverse agents interact within the system and with the system itself. The most important agents are the decision-makers. They are empowered to adopt and implement regulations, which have direct effects on other agents. If both the system and agents act in an unpredictable manner, then the probability of unexpected costs are high. Therefore, the level of predictability is a key element in evaluating the risk environment.
Arguably, the most unpredictable institutional systems are the most corrupt (Djumashev 2007; Forgues-Puccio and Okumu 2012; Goel and Ram 2013). If these systems could be placed within a continuum between fully functional rule of law and total corruption, then we could estimate the respective levels of predictability. But why should corrupt systems necessarily be unpredictable? The reason is straightforward. In heavily-corrupt systems, decision-making takes place in a nontransparent environment, where there is great uncertainty. Decision-makers possess ultimate power to favor one or another agent based on illicit payments, connections, or any other nonpublic stimulus. However, favored agents cannot be sure if some other agent will be favored at their expense in the future. Hence, foreign investors in corrupt systems face a greater probability of losing part or all of their assets. Local businesses and local society face the same probability, because corrupt systems provide tools for favoring narrow corporate or clique interests at the expense of the general public or national interest.
Risk is distinct from uncertainty, however. Institutional systems are subject to both risk and uncertainty, but uncertainty is not necessarily connected to corruption. It could well be a feature of a weak government, which is not following a straightforward strategy and is constantly bargaining with diverse groups of interests. Such behavior does not necessarily pose dangers to the interested investing party. It simply makes the decision-making system more open to interest groups. On the other hand, risk is by definition negative.[i] It is a typical feature of the corrupt system and endangers all agents within a corrupt environment.
This corrupt environment shifts the way agents behave. If rule of law is not the typical norm of behavior in the system, agents engage in informal networks of cooperation and mutual support, sometimes replacing existing formal institutions and sometimes capturing them in order to gain profit by modeling their behavior (Hellman et al. 2000; Ouzounov 2004; Jensen 2006; Yakovlev 2006; Warren 2006; Garay-Salamanca et al. 2010). These networks are often regarded as organized criminal groups, but this definition is misleading and was developed mainly for the purposes of penal law. In fact, organized crime in heavily-corrupt systems is characterized by loose organization and a strictly network character (McIllwain 1999; Finckenauer and Voronin 2001). Within the network, diverse ties with variable densities exist between the nodes. The set of nodes includes politicians, decision makers, staff of law enforcement agencies, businessmen, civil activists, criminals and other actors (Williams 2001; Morselli 2009). These networks emerge naturally in a corrupt institutional environment to handle interactions between the nodes and not necessarily to develop illegal activity. They emerge simply because public institutions do not function as regulators and must be replaced by more effective forms of regulation. In such systems, private connections replace competence, entrepreneurship and competition. Consequently, outsiders and newcomers cannot transact business without engaging in the respective networks, thus becoming part of the corrupt system of interaction.
Assessment of the level, spread and intensity of corruption and organized crime, both seen in the broader conceptual context as an environment and network of agents, respectively, is crucial for analyzing the nature of political risk. In systems where rule of law is prevalent, criminal networks are separate from the state and a clash exists between them and the law-enforcing institutions. In systems where corruption is the modus operandi of the institutional and political system, criminal networks coalesce with the state. From the foreign-investor perspective, the latter supposes much greater risk. However, this is equally relevant for local business as well, because in such uncompetitive systems monopolies emerge, and the market distorts and closes for newcomers (Gounev and Bezlov 2010; Mincheva and Gurr 2013).
Operationalization and Measurement
We have conceptualized political risk as a characteristic of a political system representing the level of corruption and spread of organized criminal networks. The more corrupt a system, the higher the political risk. This more elegant conceptualization deviates from the traditional approach to political risk analysis where corruption and organized crime are only two of many risk measures. For example, Brink (2004, 84-88) defines political risk with not only corruption and organized crime, but with political stability, political effectiveness and accountability, socio-economic conditions, internal and external conflict, military involvement in politics, religious tension, quality of bureaucracy, monetary and fiscal policy, trade policy, and public debt. However, all these metrics except for external conflict and religious tension, which could be very easily detected, are derivatives of corruption. Here we do not argue that all these factors are unimportant. Still, they could be classified as intervening variables and attributed to corruption, which in this conceptualization is the underlying factor for political risk. No corrupt system provides institutional guarantees against government arbitrariness. In corrupt systems, political effectiveness, accountability, socio-economic conditions and quality of bureaucracy are low by definition.[ii] The greater corruption is, the lower their quality. In such systems, economic and monetary policies are either irrelevant because the public interactions are regulated in an unofficial way, or are tools for greater corrupting pressure. To estimate the riskiness of a system we therefore need reliable measures of corruption and the spread of organized crime.
However, existing measures are subjective and unreliable. The Transparency International Corruption Perceptions Index is based on subjective and relatively incomparable personal analytic perceptions of the spread of corruption. The index could be used as a very general indicator, but should be interpreted with caution. International studies of the spread of organized crime do not allow even such informative systematic comparisons. The main reason for this is the nontransparent and largely illegal nature of both phenomena, which does not allow the collection of reliable data.
There are two ways to cope with low-quality data. The first is to use existing systems of measures developed by national governments for measuring the level of success of their policies for combating corruption and organized crime. However, such indicators do not exist, or at least are not publicly available. There are some national reports, which is the case in France for example, dedicated to the level of success of anti-corruption and anti-crime policies, but such reports do not provide comparable data for cross-country analysis. The second problem with this source of information is that corrupted governments are not likely to provide unbiased data.
Therefore the most realistic option remaining is the usage of indirect, yet reliable metrics to assess the level of corruption and organized crime. We offer several measures for that purpose, based on data gathered by Eurostat. Here we prefer using institutional data instead of field research, because the former are more reliable, replicable, and stable across time and space. This limits the applicability of the approach to states for which the service gathers data – i.e., Europe. But, Eurostat data are collected by national statistical agencies according to the general statistical standards applicable elsewhere in the world. To the extent that other national statistical agencies apply similar standards, the approach could be easily replicated for other continents.
Corruption Measurement
Eurostat provides data for the number of crimes recorded by the police in member states split in several categories. Given that freedom of movement of people and goods in the European Union and Schengen space is extremely easy, European countries are more or less homogenous compared to the rest of the world regarding crime levels. Therefore we can expect that if differences in crime reporting exist, these can largely be attributed to the level of public trust in law-enforcement agencies, and the level of institutionalization of law enforcement community outreach. In other words, if there are fewer reported crimes in a country relative to others, this would suggest that people do not rely on the police because they do not perceive the police as a trusted institution for handling such problems (Azfar and Murrell 2009; Hunt. 2006; Soares 2004a; Soares 2004b). Hence, lower levels of reported crime indicate higher levels of corruption, and can be used as a measure of political risk.
One inconvenience with the Eurostat data is the incommensurability of country size. This problem is solved by calculating the ratio between the number of crimes and the total population of the county for the respective year. Given that the resulting number is very small, we can standardize to produce meaningful results. Using z-scores seems a better option in comparison to the percentile rank because they allow not only for arranging countries, but for showing their relative stance to the mean, measured in standard deviations. We obtain the scores by dividing the difference between each individual ratio and the mean of the distribution by the distribution’s standard deviation.
Table 1. Number of crimes recorded by the police in 2009
Country |
Recorded offences |
Z-score |
Corruption Perceptions Index 2009 |
Sweden |
1 ,05,626 |
3.38 |
9.2 |
Belgium |
1,044,242 |
1.61 |
7.1 |
Denmark |
491,792 |
1.35 |
9.3 |
Switzerland |
676,309 |
1.31 |
9.0 |
Finland |
431,598 |
1.09 |
8.9 |
United Kingdom |
4,785,771 |
0.98 |
7.7 |
Netherlands |
1,232,482 |
0.88 |
8.9 |
Germany |
6,054,330 |
0.85 |
8.0 |
Austria |
591,597 |
0.76 |
7.9 |
Luxembourg |
32,378 |
0.59 |
8.2 |
Norway |
277,121 |
0.33 |
8.6 |
France |
3,521,256 |
0.30 |
6.9 |
Spain |
2,335,034 |
0.11 |
6.1 |
Iceland |
15,966 |
0.08 |
8.7 |
Italy |
2,629,831 |
-0.12 |
4.3 |
Slovenia |
87,465 |
-0.14 |
6.6 |
Portugal |
426,040 |
-0.24 |
5.8 |
Hungary |
394,034 |
-0.26 |
5.1 |
Estonia |
48,359 |
-0.37 |
6.6 |
Greece |
386,893 |
-0.42 |
3.8 |
Liechtenstein |
1,216 |
-0.43 |
|
Czech Republic |
332,829 |
-0.51 |
4.9 |
Poland |
1,129,577 |
-0.58 |
5.0 |
Malta |
11,953 |
-0.60 |
5.2 |
Latvia |
56,548 |
-0.73 |
4.5 |
Lithuania |
76,291 |
-0.80 |
4.9 |
Slovakia |
104,905 |
-0.91 |
4.5 |
Bulgaria |
138,105 |
-0.95 |
3.8 |
Croatia |
73,497 |
-1.00 |
4.1 |
Kosovo |
33,131 |
-1.04 |
|
Serbia |
102,369 |
-1.08 |
3.5 |
Romania |
299,889 |
-1.08 |
3.8 |
Montenegro |
8,101 |
-1.12 |
3.9 |
Cyprus |
7,104 |
-1.24 |
6.6 |
Source: Eurostat and Transparency International [02/12/2012]
Table 1 presents the Eurostat data for the total number of criminal offences recorded by police in 34 European countries in 2009. The third column of the table contains the z-scores of the ratios between the number of crimes and the total population of the country. The countries are ordered according to their z-scores. Higher scores represent lower levels of corruption. The distribution is right-skewed, which means that the bulk of countries fall below the average score of 0. The median equals -0.25. The upper quartile of the distribution is 0.78, which theoretically suggests that the top 25% of countries are scored higher. The actual share of observations in the sample scoring higher than 0.78 is 23.5%. These could be classified as countries with low levels of corruption. The lower quartile of the distribution equals -0.82 and contains about 24% of the observations. These are countries with serious problems regarding high levels of corruption. The rest of the countries fall between. Notably, Sweden has an extremely high score of 3.4 standard deviations above the mean. This could be attributed to high per capita reported crime levels alongside low corruption levels in this country.
To check the accuracy of this metric we provide the Corruption Perceptions Index (CPI) scores of Transparency International in the last column of the table. The scores theoretically range between 0 and 10. Lower scores represent higher levels of corruption. The Pearson correlation coefficient for the z-scores and CPI scores is 0.81, indicating strong positive correlation between both the index presented here, and the CPI scores. Given that the CPI is based on a sample of the population and has relatively wide confidence intervals, the true correlation could be even higher. Thus, we have a reliable measure of corruption, conceptualized as an institutional setting where agents settle their relations in an unofficial and non-institutional manner.
Market Distortion
In addition to corruption we need an indirect measure of organized crime. As organized crime aims at maximizing its economic power through state capture and resulting market distortions (Hellman et al. 2000), such a metric could be the ratio between growth of domestic demand and inflation (measured as the Harmonized Index of Consumer Prices). This ratio would reflect market distortions resulting from cartels, monopolies and/or excessive interference of the state as a result of captured economic institutions. If the price rates go well ahead of demand they are not likely to be market-driven. Table 2 presents inflation and domestic demand data for 30 European countries. Again z-scores for the ratios are presented. In the top rows of the table we see countries that were rated less favorably according to the first metric like Croatia and Cyprus. However, the general picture remains quite the same. The most risky countries, according to this measure, lie below the lower quartile of -0.61. These are economies where prices grow faster than domestic demand.
Table 2. Inflation and domestic demand in 30 European countries 2011 (2005=100)
Country |
Inflation |
Domestic demand |
Ratio |
Z-score |
Poland |
120.10 |
131.90 |
1.10 |
1.88 |
Croatia |
118.49 |
127.60 |
1.08 |
1.68 |
Sweden |
112.31 |
113.90 |
1.01 |
1.11 |
Slovakia |
116.79 |
116.30 |
1.00 |
0.94 |
Luxembourg |
117.32 |
116.30 |
0.99 |
0.90 |
Cyprus |
115.93 |
114.50 |
0.99 |
0.87 |
Germany |
111.10 |
108.90 |
0.98 |
0.80 |
Netherlands |
110.23 |
107.20 |
0.97 |
0.73 |
Norway |
113.10 |
108.50 |
0.96 |
0.61 |
France |
111.28 |
106.70 |
0.96 |
0.61 |
Finland |
114.16 |
109.10 |
0.96 |
0.58 |
Czech Republic |
116.20 |
110.40 |
0.95 |
0.53 |
Austria |
113.42 |
107.70 |
0.95 |
0.52 |
Belgium |
115.27 |
108.80 |
0.94 |
0.47 |
Malta |
115.19 |
105.00 |
0.91 |
0.18 |
Slovenia |
118.03 |
105.50 |
0.89 |
0.01 |
Denmark |
113.80 |
101.40 |
0.89 |
-0.01 |
Italy |
113.80 |
98.90 |
0.87 |
-0.21 |
Romania |
143.04 |
123.60 |
0.86 |
-0.26 |
Spain |
116.35 |
99.50 |
0.86 |
-0.34 |
Portugal |
112.72 |
95.50 |
0.85 |
-0.41 |
United Kingdom |
119.60 |
100.90 |
0.84 |
-0.44 |
Ireland |
106.60 |
89.30 |
0.84 |
-0.50 |
Lithuania |
133.90 |
105.60 |
0.79 |
-0.94 |
Bulgaria |
141.21 |
106.30 |
0.75 |
-1.27 |
Estonia |
133.40 |
100.40 |
0.75 |
-1.27 |
Greece |
121.35 |
91.30 |
0.75 |
-1.28 |
Iceland |
159.22 |
117.10 |
0.74 |
-1.43 |
Latvia |
143.73 |
96.60 |
0.67 |
-2.01 |
Hungary |
134.79 |
89.90 |
0.67 |
-2.05 |
Source: Eurostat [02/12/2012]
Shadow Economy
Finally, the spread of the shadow economy could be indirectly measured by the difference between annual net earnings and the actual consumption of the population. Small differences would suggest that there are more informal earnings, and therefore the shadow economy is large. Table 3 presents the data, together with z-scores. The distribution is clearly bi-modal. There are two distinctive clusters of countries. The first group has very high differences while in the second those differences are minimal. The top 25% are one standard deviation above the mean of 0 (Q3=1). The lower quartile equals -0.93 and contains 23% of the sample countries.
Table 3. Earnings and consumption in 30 European countries 2010
Country |
Annual net earnings |
Actual individual consumption |
Difference |
Z-score |
Belgium | 53,871 | 22,400 | 31,471 |
1.67 |
Germany | 50,594 | 21,300 | 29,294 |
1.44 |
Norway | 66,128 | 37,200 | 28,928 |
1.41 |
Netherlands | 49,919 | 22,200 | 27,719 |
1.28 |
France | 49,593 | 22,200 | 27,393 |
1.25 |
Austria | 49,719 | 22,600 | 27,119 |
1.22 |
Sweden | 50,737 | 25,300 | 25,437 |
1.05 |
Finland | 48,898 | 24,000 | 24,898 |
1.00 |
Switzerland | 57,877 | 34,200 | 23,677 |
0.87 |
United Kingdom | 44,245 | 21,600 | 22,645 |
0.77 |
Luxembourg | 54,993 | 33,200 | 21,793 |
0.68 |
Denmark | 50,498 | 29,600 | 20,898 |
0.59 |
Italy | 37,278 | 18,800 | 18,478 |
0.34 |
Spain | 32,197 | 16,000 | 16,197 |
0.11 |
Iceland | 35,275 | 20,500 | 14,775 |
-0.03 |
Ireland | 35,781 | 22,000 | 13,781 |
-0.13 |
Greece | 26,197 | 15,900 | 10,297 |
-0.49 |
Portugal | 21,473 | 12,700 | 8,773 |
-0.64 |
Malta | 19,218 | 10,600 | 8,618 |
-0.66 |
Slovenia | 19,638 | 12,100 | 7,538 |
-0.77 |
Turkey | 12,640 | 5,400 | 7,240 |
-0.80 |
Czech Republic | 15,227 | 8,800 | 6,427 |
-0.88 |
Estonia | 12,876 | 6,800 | 6,076 |
-0.92 |
Hungary | 11,717 | 6,200 | 5,517 |
-0.98 |
Latvia | 10,191 | 6,100 | 4,091 |
-1.12 |
Poland | 10,379 | 6,600 | 3,779 |
-1.15 |
Slovakia | 11,768 | 8,200 | 3,568 |
-1.17 |
Romania | 7,134 | 4,200 | 2,934 |
-1.24 |
Lithuania | 8,878 | 6,300 | 2,578 |
-1.27 |
Bulgaria | 4,551 | 3,400 | 1,151 |
-1.42 |
Source: Eurostat [02/12/2012]
Political Risk Index
If we combine the three measures we create an alternative index of political risk, based on the concept that the most corrupt, distorted, and shadowy institutional systems are the most risky. The three metrics could be assigned different weights, but given that they are indirect and could reflect other phenomena apart from those for which they are introduced, we assign equal weights to improve precision.
Note that there are data on some measures for some countries, but on others data is missing or time differs. However, the operational definition of corruption is not particularly time-sensitive. Therefore combining the scores improves precision and fixes issues of missing data. Table 4 presents the risk index for the countries for which we obtained data. Prior to averaging the scores of the three metrics they were normalized to fall within the range of 0 to 1. A score of 1 indicates high political risk, and a score of 0 indicates no political risk. Note that the scale is inversed in comparison with the scales of the three initial metrics. This is intended to make the index more intuitive – higher scores indicate higher levels of political risk. The index arranges the countries relative to one another. The separate values would change if more cases are added to the sample.
Table 4. Political Risk rating of 27 countries, 2011
Country |
Political Risk Index |
Corruption Perceptions Index 2011 |
World Bank Control of Corruption Index |
World Bank Government Effectiveness Index |
Coface Rating 2008-2009 |
Latvia | 0.94 | 4.2 | 0.21 | 0.68 | A3 |
Bulgaria | 0.92 | 3.3 | -0.17 | 0.01 | A4 |
Hungary | 0.89 | 4.6 | 0.34 | 0.71 | A3 |
Lithuania | 0.87 | 4.8 | 0.29 | 0.68 | A3 |
Estonia | 0.83 | 6.4 | 0.91 | 1.2 | A2 |
Romania | 0.83 | 3.6 | -0.2 | -0.22 | A4 |
Greece | 0.78 | 3.4 | 0.93 | 0.99 | A2 |
Slovakia | 0.71 | 4 | 0.29 | 0.86 | A3 |
Iceland | 0.71 | 8.3 | 1.94 | 1.57 | A1 |
Portugal | 0.71 | 6.1 | 1.09 | 0.97 | A2 |
Malta | 0.69 | 5.6 | 0.91 | 1.16 | A2 |
Czech Republic | 0.68 | 4.4 | 0.32 | 1.02 | A2 |
Slovenia | 0.68 | 5.9 | -0.15 | 0.48 | A1 |
Spain | 0.6 | 6.2 | 1.06 | 1.02 | A1 |
Poland | 0.6 | 5.5 | 0.51 | 0.68 | A3 |
Italy | 0.58 | 3.9 | -0.01 | 0.45 | A2 |
United Kingdom | 0.47 | 7.8 | 1.54 | 1.55 | A1 |
Denmark | 0.43 | 9.4 | 2.42 | 2.17 | A1 |
Luxembourg | 0.4 | 8.5 | 2.17 | 1.73 | A1 |
France | 0.38 | 7 | 1.51 | 1.36 | A1 |
Austria | 0.36 | 7.8 | 1.44 | 1.66 | A1 |
Norway | 0.36 | 9 | 2.17 | 1.76 | A1 |
Finland | 0.35 | 9.4 | 2.19 | 2.25 | A1 |
Netherlands | 0.32 | 8.9 | 2.17 | 1.79 | A1 |
Germany | 0.3 | 8 | 1.69 | 1.53 | A1 |
Belgium | 0.25 | 7.5 | 1.58 | 1.67 | A1 |
Sweden | 0.13 | 9.3 | 2.22 | 1.96 | A1 |
Sources: CPI is excerpted from www.transparency.org [02/12/2012]. Coface ratings are excerpted from The Handbook of Country Risk 2008: A Guide to International Business and Trade. London: GMB 2008. WB scores are excerpted from the Worldwide Governance Indicators database (http://info.worldbank.org/governance/wgi/) [02/12/2012].
The scores of Transparency International CPI, World Bank indices for control of corruption and government effectiveness, as well as the Coface Country @rating are presented for comparison. The rating of Coface is based on letter grades ranging from A to D, where D represents the highest risk. The A grade has 4 subcategories. The grade is based on assessment of three dimensions: economic, political and financial outlook. The two World Bank indices range between -2.5 and 2.5. Lower scores represent higher corruption. The table shows that the rankings are comparable. The correlation coefficient between our index and the CPI is -0.81, indicating strong inverse correlation (higher scores on our index indicate higher risk while on CPI – less corruption). Correlations with the two World Bank indices are both -0.78. The countries rated in the lower rows of the table are graded A1 by Coface. However, our index provides a more differentiated rating than Coface, which grades 14 of the 27 countries with A1.
The index we introduce proves to be reliable and provides a fine-grained cross-country comparison. The index produces a split of the countries into two clusters. The first represents the group of risky countries, with scores between 1 and 0.6. The low-risk countries fall in the second cluster with scores ranging from 0.5 to 0.1 (Figure 2). The index is based on institutional statistical data which makes it more objective than other indices. It allows reliability in time and cross-country comparison. What is most important, however, is the possibility for differentiation among the developed countries, which is not possible with the existing risk indices.
Conclusion
In this paper we offer an alternative conceptualization of political risk, based on corruption, market distortion, and the shadow economy. Within a continuum between rule of law and corruption, the levels of political risk vary greatly. The results prove to be highly reliable, in that they are correlated with existing indices. However, they improve on them as our index is not based on subjective personal judgments and is much more precise in differentiating countries. The index can easily be replicated in time and space.
The author is Assistant Professor at the Political Science Department, “St. Kliment Ohridski” University of Sofia, Bulgaria.
References
Azfar, Omar and Peter Murrell. 2009. “Identifying Reticent Respondents: Assessing the Quality of Survey Data on Corruption and Values” Economic Development and Cultural Change, 57/2, 387-411.
Bouchet, Michel H., Ephraim Clark and Bertrand Groslambert. 2003. Country Risk Assessment: A Guide to Global Investment Strategy. Wiley.
Brink, Charlotte H. 2004. Measuring Political Risk: Risks to Foreign Investment. Aldershot: Ashgate Publishing Ltd.
Djumashev, Ratbeg. 2007. “Corruption, Uncertainty and Growth.” Monash Economics Working Papers #15-07.
Feils, Dorothee J. and Florin M. Sabac. 2000. “The Impact of Political Risk on the Foreign Direct Investment Decision: A Capital Budgeting Analysis.” Engineering Economist, 45/2, 129–43.
Finckenauer, James O. and Yuri A. Voronin. 2001. The Threat of Russian Organized Crime. US Department of Justice: National Institute of Justice.
Forgues-Puccio, Gonzalo F. and Ibrahim Okumu. 2012. “Does Size Matter? Scale, Corruption and Uncertainty.” CDMA Working Paper Series #1207.
Garay-Salamanca, Luis J., Eduardo Salsedo-Albaran and Isaac De Leon-Beltran. 2010. Illicit Networks Reconfiguring States: Social Network Analysis of Colombian and Mexican Cases. Bogota: Metodo Foundation.
Goel, Rajeev K. and Rati Ram. 2013. “Economic Uncertainty and Corruption: Evidence from a Large Cross-Country Data Set” Applied Economics, 45/24, 3462-3468.
Gounev, Philip and Tihomir Bezlov. 2010. Examining the Links between Organized Crime and Corruption. Sofia: Center for the Study of Democracy.
Hellman, Joel S., Geraint Jones and Daniel Kaufmann. 2000. “Seize the State, Seize the Day: State Capture, Corruption and Influence in Transition.” World Bank Policy Research Working Paper Series #2444.
Howell, Llewellyn D. 2001. Defining and Operationalizing Political Risk in Llewellyn D. Howell (ed.) Political Risk Assessment: Concept. Method and Management. The PRS Group Inc.
Howell, Llewellyn D. and Brad Chaddick. 1994. “Models of Political Risk for Foreign Investment and Trade: An Assessment of Three Approaches.” Columbia Journal of World Business 29/3, 70–91.
Hunt, Jennifer. 2006. “How Corruption Hits People When They Are Down.” NBER Working Paper #12490, Cambridge, MA: National Bureau of Economic Research.
Jensen, Nathan M. 2007. “Economic Reform, State Capture, and International Investment in Transition Economies.” Comparative Political Studies, 36/9, 1092-1111.
Kobrin, Stephen J. 1979. “Political Risk: A Review and Reconsideration.” Journal of International Business Studies, 10/1, 67–80.
McIllwain, Jeffrey S. 1999. “Organized Crime: A Social Network Approach.” Crime, Law and Social Change, 32/4, 301-323.
McKellar, Robert. 2010. A Short Guide to Political Risk. Farnham: Gower Publishing Ltd.
Meldrum, Duncan H. 2000. “Country Risk and Foreign Direct Investment.” Business Economics, 35/1, 33–40.
Mincheva, Lyubov G. and Ted R. Gurr. 2013. Crime-terror Alliances and the State: Ethnonationalis and Islamist Challenges to Regional Security. Routledge.
Morselli, Carlo. 2009. Inside Criminal Networks. Springer.
Ouzounov, Nikolay A. 2004. “Facing the Challenge: Corruption, State Capture and the Role of Multinational Business.” The John Marshall Law Review, 37/4, 1181-1204.
Robock, Stefan H. 1971. “Political Risk: Identification and Assessment.” Columbia Journal of World Business, 6/4, 6–20.
Root, Franklin R. 1972. Analyzing Political Risks in International Business. In: Ashok Kapoor and Phillip D. Grub (eds.) The Multinational Enterprise in Transition. Princeton: Darwin Press, 354–65.
Roy, Ashok and Patricia G. Roy. 1994. “Despite Past Debacles, Predicting Sovereign Risk Still Presents Problems.” Commercial Lending Review, 9/3, 92–5.
Simon, Jeffrey D. 1982. “Political Risk Assessment: Past Trends and Future Prospects.” Columbia Journal of World Business, 17/3, 62–70.
Soares, Rodrigo R. 2004a. “Crime Reporting As A Measure of Institutional Development” Economic Development and Cultural Change, 52/4, 851-871.
Soares, Rodrigo R. 2004b. “Development, Crime and Punishment: Accounting For the International Differences In Crime Rates” Journal of Development Economics 73/1, 155-184.
Warren, Mark E. 2006. “Political Corruption as Duplicitous Exclusion.” Political Science and Politics, 39/4, 803-807.
Williams, Phil. 2001. Transnational Criminal Networks. In: John Arqula and David Ronfeld (eds.) Networks and Netwars: The Future of Terror, Crime and Militancy. Santa Monica: RAND Corporation.
Yakovlev, Andrei. 2006. “The Evolution of Business: State Interaction in Russia: From State Capture to Business Capture?” Europe-Asia Studies, 58/7, 1033-1056.
[i] For an alternative interpretation see Robock (1971), Kobrin (1979), Feils and Sabac (2000).
[ii] Note that political instability that inflicts harm on investors and the public in general could occur in less corrupt countries. Such is the case with the relatively high probability of terrorist attacks in some well-developed western countries. We should however distinguish between two types of terrorist threats. The first one is external and stems from foreign policy. Such is the case with Al-Qaeda attacks in the US and other parts of the world. Given the fact that these attacks are confronted by the massive power and counteraction of the state, their probability is very small. Even if they occur, their effect is limited in time and space. The second type of terrorist risk is imposed by local organizations seeking political goals such as the Irish Republican Army (IRA) and the Basque Homeland and Freedom organization (ETA). Local terrorism proves to be much more frequent and inflicts more damage to the business climate.
JPR Working Paper. Peer Review Status: 2/3.